Beyond Data Literacy: Helping Students Achieve Data Fluency Through Collaboration and Real-World Applications
Beyond Data Literacy: Helping Students Achieve Data Fluency Through Collaboration and Real-World Applications
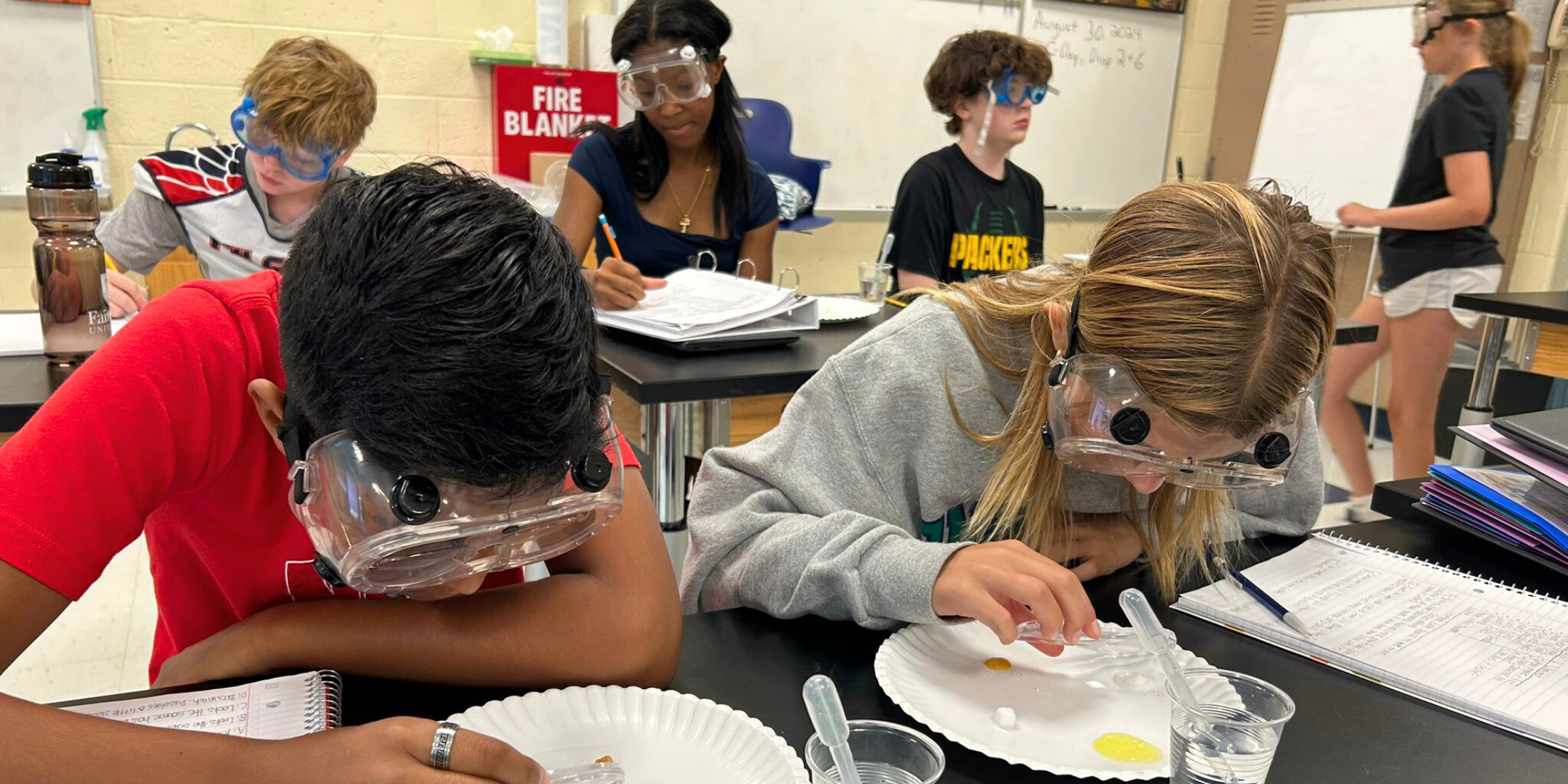
Quick Take: In today’s data-driven world, preparing students for the future workforce requires more than just data literacy—it demands data fluency. This blog explores how Collaborate Data Analysis and real-world applications help students go beyond basic data skills to develop the critical thinking and problem-solving abilities needed to thrive in modern industries. Using examples from our K-12 curricula, including OpenSciEd, discover how to seamlessly integrate data fluency into your classroom and equip students with the tools they need for success in a data-rich future.
Why Are Educators Across the Country Sounding the Alarm About Data Literacy?
Imagine a classroom where every lesson, regardless of subject, equips students with the ability to interpret and utilize data—skills rapidly becoming as fundamental as reading and writing. Earlier this year, five teacher associations representing mathematics (NCTM) (ASA), science (NSTA), social studies (NCSS), and technology (CSTA) released a joint call for data science to be taught across all grades and academic subjects. Educators nationwide recognize the critical need to empower students with essential data skills that will prepare them for the future.
But why this sudden urgency?
The answer lies in the rapidly evolving landscape of our workforce, emerging technologies, and society at large. As artificial intelligence and machine learning technologies become increasingly integrated into all sectors (including the sciences), understanding and working with data has never been more critical. Data is the new currency that powers industries, drives innovation, and influences every aspect of our lives. Data skills are no longer the exclusive domain of statisticians and data scientists – they’re becoming essential tools for everyone.
A recent Forrester study revealed that 82% of decision-makers expect at least basic data literacy from all employees in their departments. Every employee must understand and work with data in their day-to-day roles. Data analysis is one of the most in-demand skills in the modern workforce.
Advancing Data Science and Literacy Programs in Schools
According to a recent article in EdWeek, the urgency to enhance data science education is being met head-on by states nationwide. Currently, 27 states are taking official steps to advance data science education. Nineteen states have established subject codes at the state level, facilitating the implementation of data science and literacy programs in schools and districts. Five states have gone further, adopting new standards that emphasize data literacy and science foundations. There's increasing urgency at the federal level, too, with a bipartisan data science literacy act introduced in the House in 2023 and ongoing discussions in the Senate.
This movement isn’t just about adding a new subject to the curriculum; it’s about transforming how we teach and learn about data. Educators are rallying together in a powerful collective effort—not because industry leaders demand it, but because they see firsthand the importance of data literacy in preparing students to succeed in an increasingly AI-driven society. The ability to read, understand, interpret, and communicate data effectively is now as essential as reading and writing.
Beyond traditional data literacy education lies a new, innovative, and profoundly effective method for teaching data science, one that aligns with the principles outlined by leading teaching associations. This post explores how Collaborative Data Analysis allows science educators to seamlessly teach data literacy while elevating students from data literacy to data fluency. We’ll illustrate this through examples from OpenSciEd curriculum units.
Challenges Teaching Data Literacy the Traditional Way
Teachers today often use a structured approach to teaching data science, walking students through reading and interpreting graphs, including identifying what's data, understanding units, and analyzing color coding.
But, as the demand for data skills surges, our traditional approaches to teaching data literacy, like standalone lessons on how to read graphs or calculate basic statistics, are falling far short. These outdated methods fail to engage students and prepare them for the complex, data-rich world they'll enter after graduation.
Adding to this challenge is the significant burden placed on teachers. Many teachers lack access to comprehensive, standards-aligned instructional materials that seamlessly integrate data skills into their existing curricula. Without these resources, teachers are often left to create their own materials, which can be time-consuming. Moreover, there's a significant disconnect between what students learn in school and the skills required in today's data-driven workforce.
Traditional teaching methods often don't mirror the collaborative, team-based data processing that is standard in many industries. This misalignment between classroom learning and real-world needs could be devasting for students entering the workforce. While schools often focus on teaching isolated skills, industries are desperately seeking employees who can strategically apply data insights strategically across various contexts.
As we grapple with these challenges, it becomes increasingly clear that a new approach is needed to bridge the gap between classroom learning and real-world application, alleviate the burden on teachers, and equip students with the collaborative, critical thinking skills they need to succeed in a data-driven world.
Making the Leap from Data Literacy to Data Fluency
Data literacy and data fluency represent different levels of data competency, with fluency being a more advanced and comprehensive skill set.
While data literacy equips students with the foundational ability to read, understand, and work with data, data fluency takes this a step further by fostering proficiency in interpreting, communicating, and applying data insights across various contexts. This distinction is crucial in today's data-driven world, where the ability to not only comprehend data but also to leverage it strategically is highly valued. As illustrated in the graphic below, illustrating the key differences between these two concepts, data fluency encompasses a broader and more dynamic skill set compared to data literacy.
Data literacy involves basic statistical concepts, the ability to interpret simple graphs, recognize data sources, perform basic data analysis, and maintain data privacy awareness. These skills provide students with a foundational understanding, enabling them to handle data at an entry level.
On the other hand, data fluency includes advanced data interpretation, effective communication of data insights, creative problem-solving with data, and the ability to generate actionable insights. It's about developing a deep, intuitive understanding of data that allows students to interpret, analyze, and apply data insights creatively across various contexts.
Data fluent students can ask the right questions, identify patterns, challenge assumptions, and turn raw data into actionable information. This level of competency is what industries increasingly demand and what students need to thrive in our data-driven world.
However, much of today’s data science education remains confined to data literacy. Science teachers have a unique and powerful opportunity to embrace data fluency and to elevate students to the new standard in a way that decreases the burden of teaching traditional data science literacy.
Collaborative Data Analysis: An ‘Application-First’ Way to Teach Data Science
In their joint call for enhanced data science education, five major teacher associations emphasized data science's interdisciplinary, inquiry-based, and collaborative nature. They highlighted how data science uses complex methods to explain trends, encouraging students to ask compelling questions, investigate data, analyze results, and communicate their findings effectively.
Their vision of effective data science education aligns with our K-12 award-winning STEM curricula, which use an innovative Collaborative Data Analysis approach to teaching modern data skills. Rather than isolating data skills in standalone lessons, our STEM programs integrate data science seamlessly into curriculum storylines. This ensures students engage with data in context-rich scenarios, making learning more engaging and interdisciplinary while reflecting how data science operates in the real world.
Collaborative Data Analysis is more than a teaching method; it’s a transformative, standards-based approach that bridges the gap between data literacy and fluency, empowering students to apply data skills creatively and strategically—building the precise competencies the modern workforce demands.
The graphic below illustrates how Collaborative Data Analysis fosters data literacy that ultimately builds data fluency.
- Collaborative Data Analysis serves as the foundation where students work together on data-driven projects, emphasizing an “Application-First" mindset. This collective engagement cultivates a shared proficiency in interpreting and analyzing data, creating a supportive learning environment where data fluency thrives.
- Through this process, students develop Individual Data Literacy, gaining the proficiency to understand, read, and apply data on their own. By working together in a collaborative environment, students acquire the essential skills needed to handle data effectively and independently.
- As students build individual data literacy, this naturally leads to Classroom-Wide Data Fluency, where students collectively reach a higher level of competency. With fluency, students can communicate insights, solve complex problems using data, and leverage their skills across various contexts, creating a classroom environment where data is understood and used strategically by all.
Collaborative Data Analysis bridges the gap between data literacy and fluency, equipping students to apply their skills in diverse, real-world scenarios. This approach aligns seamlessly with the Next Generation Science Standards (NGSS), particularly Practices 4 and 5, which focus on analyzing and interpreting data and using mathematics and computational thinking. Embedding these practices into every lesson ensures that students meet educational standards and foster a deeper understanding of scientific concepts.
This method also eases the burden on teachers by providing comprehensive, ready-to-use materials that integrate data skills into existing science curricula. Instead of designing separate data lessons, teachers can leverage structured activities that promote meaningful engagement with data.
Let's explore examples of Collaborative Data Analysis in NGSS-aligned OpenSciEd curriculum units and see how it drives data fluency in practice!
Building Data Fluency with OpenSciEd: Examples from Curriculum Units
To illustrate how OpenSciEd’s Collaborative Data Analysis approach translates into tangible classroom practices—and alleviates the burden on educators to teach data separately—let’s explore specific middle and high school curriculum units. These examples highlight how data is seamlessly integrated throughout, connecting scientific phenomena with real-world applications.
In the middle school 6.2 Thermal Energy Unit, students construct data early on, engaging with qualitative methods such as notice-and-wonder charts. Data analysis is woven into every lesson, and teachers use background resources to help students develop their skills, aligning with NGSS Practices 4 and 5.
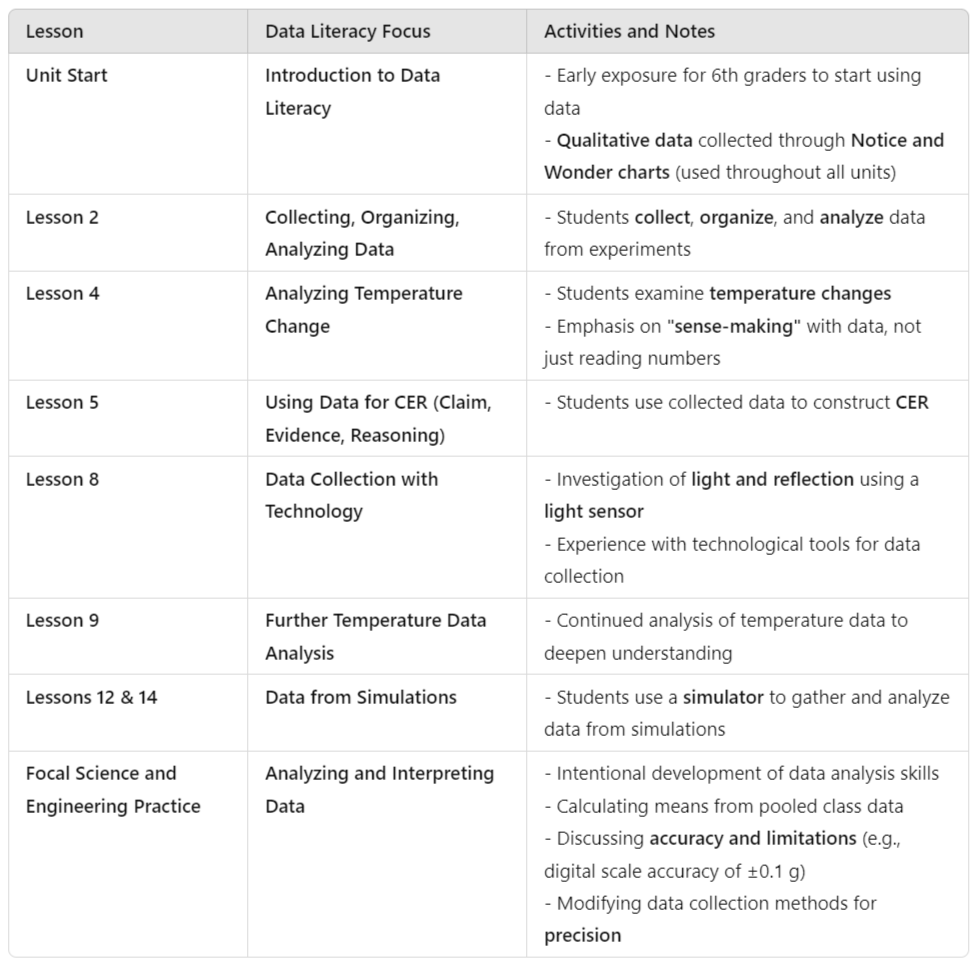
Throughout the unit, teachers utilize background knowledge resources to support students in developing their data analysis capabilities, aligning with NGSS Practices 4 and 5.
Transitioning to high school, the P.1 Energy Flow and Earth Systems Unit builds on the foundational data skills learned in earlier units. Here, students engage in more sophisticated data analysis, applying statistical concepts and computational thinking to interpret complex data sets.
The OpenSciEd high school content also includes a simulation library with data excursions powered by CODAP, a free educational software for data analysis. The simulations offer data visualization tools and interactive data sets to support student learning.
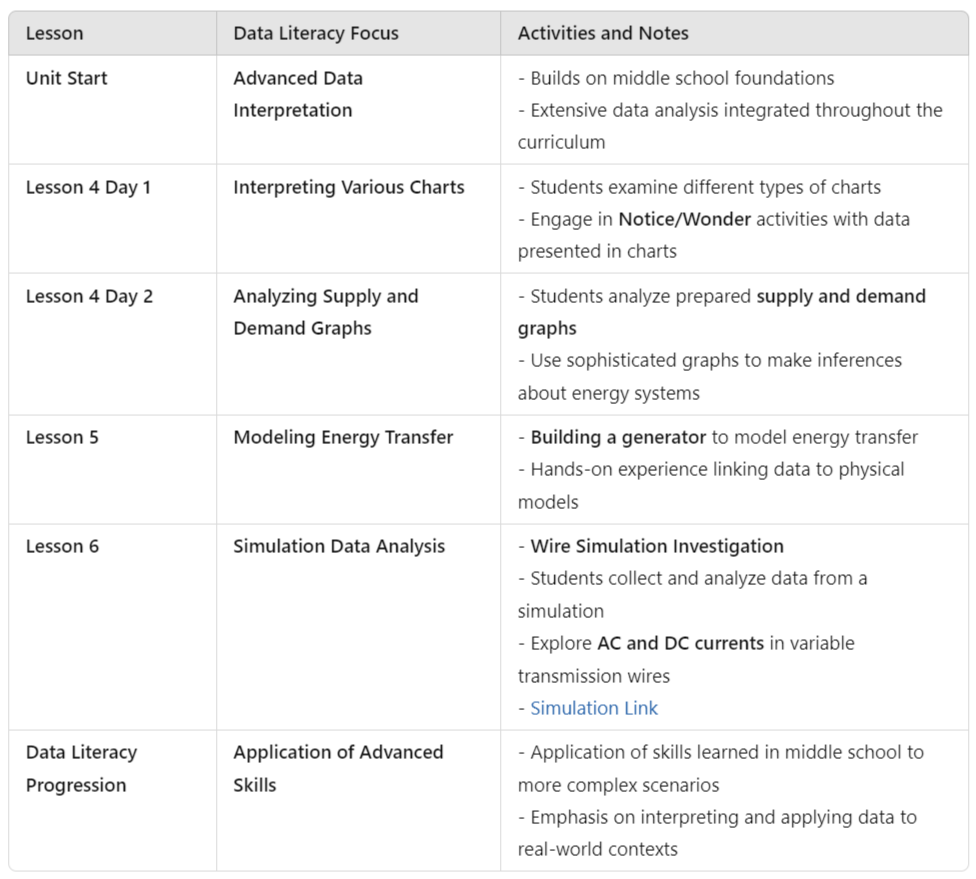
In both units, data is not simply a topic to study—it’s a tool for exploring scientific phenomena. Students engage in advanced data interpretation, critical thinking, and generating actionable insights.
The progression from middle to high school demonstrates how OpenSciEd builds data fluency over time. Students advance from basic data collection to model-based analysis using statistical techniques. Throughout, the focus remains on Collaborative Data Analysis, fostering deeper learning and an intuitive understanding of data.
This collective fluency creates an equitable learning environment where every student can effectively communicate and apply data insights. By embedding these practices into both middle and high school units, OpenSciEd ensures progressive skill development that meets—and often exceeds—NGSS standards, preparing students for real-world scientific and engineering challenges.
Empowering the Data-Fluent Generation
As we've explored, integrating meaningful data science education is more important than ever for today’s students. The call from five major teaching associations to embed data science across all grades and subjects is urgent in a world increasingly driven by AI and data.
By adopting Collaborative Data Analysis, we’re not simply teaching isolated skills—we’re empowering students to become critical thinkers and informed decision-makers, capable of applying data across disciplines and meeting the demands of a data-driven workforce.
Our K-12 curricula, including OpenSciEd, directly respond to this call by embedding data fluency into every lesson. Students learn to analyze, interpret, and apply data in ways that mirror real-world applications.
Educators now have the tools to shape a truly data-fluent generation. Let’s answer this urgent call and prepare students everywhere for a future where data fluency is not just important, but essential.
Learn more about OpenSciEd: LEARN MORE
* * * * * *